Funding Strategies for Data-Intensive Science
In the early 1990s, NASA was ready to fund a project that would generate 15 TB of images of the night sky. This was a massive amount of data for the time. It was the beginning of the first era of big data in science, where scientists and funders could invest in big projects to collect large data sets for many people to use.
But actually using that data required more than just the telescopes that NASA could fund. The data needed to be stored, curated, retrieved, and analyzed. The Alfred P. Sloan Foundation — a private philanthropy — contributed not only to the telescopes, but also contributed successive grants for the open data release, community, infrastructure, and management that was needed to turn the massive dataset of images into something transformative for astronomers.
This kind of data-intensive science (DIS) advanced not just astronomy, but also computer science and core software tools for scientific database management and data analysis. Microsoft computer scientist Jim Gray, who led the database software work of the Sloan Digital Sky Survey, was a strong advocate for it. Gray recognized that DIS is a fundamental evolution of the scientific method: scientific innovation, as a process, is radically altered by massive scale compute and large, complex datasets.
We are poised for an explosion in scientific discovery through DIS. Across a wide range of scientific disciplines, the cost of data generation is dropping. Data generation now leverages cheap sensors, robotics and automation, which drive smaller, cheaper, and higher throughput methods to make data. At the same time, the cost of data storage and processing also continues to decline. As a result, we can now create datasets of ever increasing size at lower and lower costs, and connect them to larger and larger models built through machine learning. We’re entering a world in which large, well-curated datasets enable us to predict scientific outcomes computationally before we test them empirically.
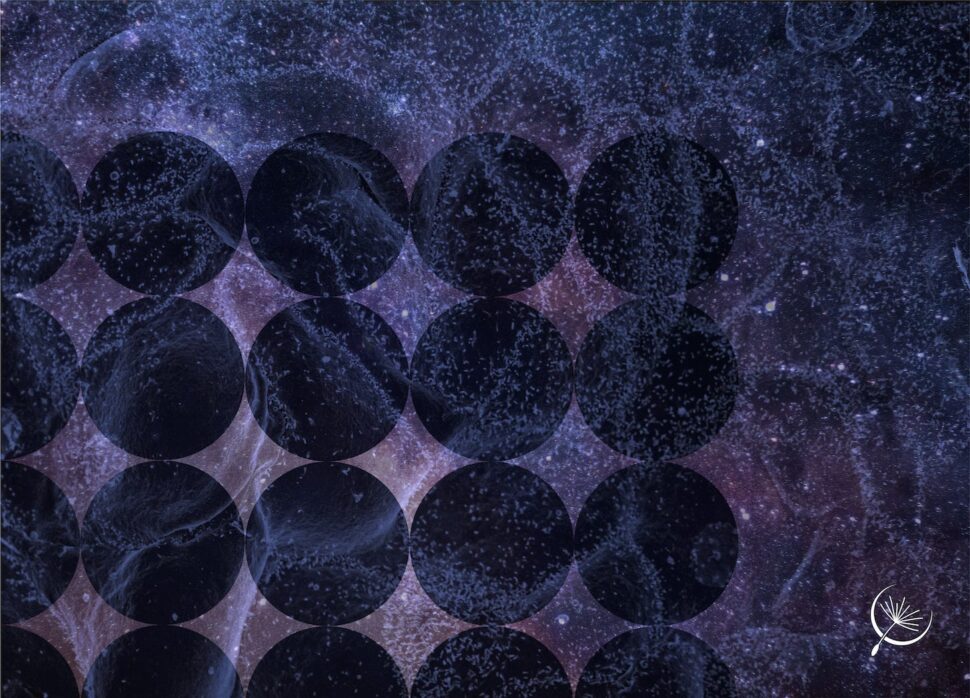
We’re not ready.
Without a change in how we organize scientific research itself, we won’t be able to use these developments in data generation and modeling at scale. Science is often organized around the laboratory, the principal investigator, and the publication, rather than data, software, and computational power. This approach creates different cultures between institutions and scientific disciplines, and affects their usage of computation, collaboration, and ability to scale.
New ways of performing science with data at a massive scale will look quite different from the ‘one lab, one hypothesis’ experiments we are familiar with. Gray’s work on the Sloan Digital Sky survey intentionally separated data capture, data curation, and data analysis into distinct categories with their own funding, technology, labor forces, incentives, and goals. These distinct categories accelerated the team’s capabilities to fundamentally accelerate astronomical discoveries.
DIS goes far beyond simply increasing the scale of data generated. This is illustrated by the Human Genome Project and the Mars Rovers missions. While they might seem fundamentally different, these initiatives shared starting goals of obtaining more data at a scale that seemed impossible. And each quickly arrived at the same conclusion: more data means scientists need to perceive data and work with that data through software, which requires more processors, more metadata, more software engineers — and more collaboration.
And while the infrastructure for data creation, storage, and analysis is exponentially growing, the cultural infrastructure of how we fund and collaborate in science hasn’t kept pace. There is a colossal opportunity to test novel systems to support the scientific research process — systems that integrate rapidly scaling technologies.
It’s also an opportunity that requires scientists to be better at collaborating with each other in their day to day work. The systems that drive science today frequently reward single investigators running laboratories at academic institutions, who receive tenure and funding based on their publication metrics in elite scholarly journals. Those systems, which sit at the heart of so much American science, combine data capture, curation, and analysis, keeping all three inside the same labs, labor forces, and technological environments. Combining these activities cuts against the collaborations required for DIS; it’s partly why even data projects essential to their fields can result in conflict, rather than collaboration.
The opportunity is ripe for funders of science to invest in new systems of data capture, curation, and analysis that are built on the foundation of DIS. These new kinds of investments will generate public data goods that could speed up the pace of scientific discoveries in measurable ways. We envision this data-centric way of doing and funding science as an iterative system: data is funded through feedback from scientists and published as a core good for many scientists, who then form networks of users. Their needs then inform the next iteration of core data goods that receive funding.
This is far easier said than done. The cost of data generation is still high, even with trends driving it down. Cloud computing has costs that bite, especially when compared to the costs of using local machines that are often hidden in overhead. This leads to questions about capital constraints: How do funders make smart choices about capital deployed to support DIS? Where does data live over the long term? How can funders validate that users are actually addressing their scientific problems at hand? We have to rethink what success looks like in scientific funding, not just pay for data at scale.
Falling in love with problems
Many champions have hailed the value of generating and sharing large amounts of data to advance science. The Open Science movement, of which I have enthusiastically been a part of for two decades, has advocated for opening up datasets generated by the traditional infrastructures of science that follow a ‘one lab, one problem, one dataset, one grant’ approach. While the movement has very successfully changed government policies across the world to open up this data, relying on individual labs working towards individual papers has led to a gulf between data creators and data users.
Data curation, our best bet to close that gulf, is rarely funded in ways that are adequate to enable DIS to emerge. Data publishing has been siloed, has led to repository proliferation, and has created data discovery problems. And data at scale is often simply too expensive to download and upload for systems of collaboration that require copying and redistributing data to work. As a result, DIS is mostly happening at the edges of many sciences, and not at the center for funders or research institutions.
In contrast, DIS succeeds to the extent that it allows the exploration of many solutions by many people across many points in time. Instead of asking “How does this data solve this specific problem?” we ask “How does this data increase our ability to create new models and predictions across a wide range of inquiry?” In many ways, this looks a lot more like a software product approach — it’s an iterative, human-centered process to test and validate hypotheses around proposed solutions.
The central concept of iteration — characterized by rapid prototyping, frequent testing, incremental improvements, and user feedback loops — can significantly reshape how large scientific datasets are generated and funded. Funders could build projects that release early MVP datasets to validate experimental methods, explore how much data is truly needed at scale, and refine metadata. This iterative approach reduces upfront risk and improves outcomes through early feedback. It also allows us to build modular datasets that allow new data layers to be added over time as data generation technology evolves.
DIS puts more responsibility on the funder of science to be creative partners with scientists. Funders have the scale, leverage, and incentives to generate data and measure its impact beyond what an individual lab can do. But that capacity also needs to come with the recognition that most scientists don’t live in a data-intensive system, and therefore don’t have the time, funding, and resources to build DIS systems without meaningful change. There’s a huge opportunity for funders to close that gap by devoting time and resources to the systems that enable DIS.
Doing the experiment
At Astera, one of our tenets is “Do the experiment.” A concrete example of how we’re trying to implement this iterative approach in our data program at Astera is in our microbial data work. Rather than starting by negotiating five years of data generation, curation, and analysis with a bunch of long-term research grants to elite academic institutions, we’re probing the field with a request for information, talking to the scientists who responded (currently underway), and looking to build a data MVP through a contract research structure.
We’ll then use that MVP to help understand what data is actually most informative for scientists trying to predict microbial phenotype from microbial genotype: is it tens of thousands of microbes with a little bit of data each? Is it a few hundred microbes with an exhaustive amount of data each? Is it somewhere in between? What can we learn about the individual data types if we experiment with knocking rows and columns out of the data product? How much data does it take to make predictions more accurate? Most importantly — what does this do for scientific discovery, and how can we use that information to inform our decisions to scale the data over time?
I’ve long been an advocate of open science systems. But as a funder I’ve translated that into a strong belief in DIS investments — with particular emphasis on data generation and curation. This move has come with a shift in mindset that holds great potential for the field of scientific discovery. It obliges us to think differently than we would with a traditional grantmaking approach, which is often optimized for the ‘one-lab, one grant’ system. It is time to invest in data goods that are built iteratively from the beginning to create value for many users, support deep curation to empower them to solve many problems, and drive model building to enable predictive science. This is how we get closer to our overall goal of accelerating the pace of and capacity for scientific discovery.